History and Basic Concepts of Molecular Modeling
- Ege Altun
- Apr 28
- 5 min read
Updated: Jun 10
What is Molecular Modeling?
Molecular modeling is a technique that uses computer-aided methods to understand, predict, analyze or visualize the physical and chemical properties of atoms and molecules. It is of great importance especially in areas such as drug design, materials science and biotechnology.
Historical Development of Molecular Modeling
Molecular modeling laid its foundations in the 1920s and 1930s with the development of quantum mechanics. The work of scientists such as Erwin Schrödinger and Werner Heisenberg provided a theoretical framework for understanding the behavior of atoms and molecules. The Schrödinger equation made it possible to calculate bond structures and molecular energies by predicting how electrons behave in a molecule.
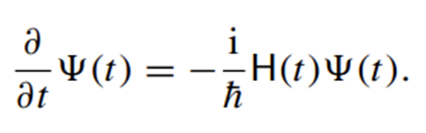
In the 1940s, studies were conducted to analyze molecular structures using Newtonian mechanics. During this period, theoretical models were developed to calculate bond lengths and angles, steric interactions (repulsive forces caused by the physical proximity of atoms) and energy minima (the most stable and low-energy state of a system) were investigated. These studies, which investigated the energetic effects of structural changes in molecules, formed the basis of modern molecular modeling techniques (2).
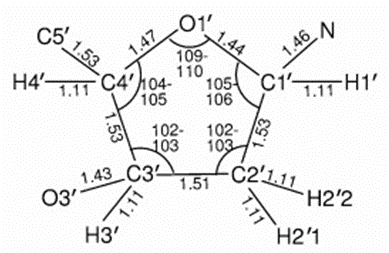
In 1953, the foundations of modern simulation techniques were laid by developing Monte Carlo methods and the Metropolis algorithm (4). In 1957, Alder and Wainwright performed the first molecular dynamics simulation, known as the "Hard Sphere" model (5). In the 1960s, computers began to be used in the calculation of force fields and algorithms were developed for conformational analysis. In 1971, the Protein Data Bank (PDB) (6) was established and the first protein dynamics simulation was performed in 1977 (7). In the 1980s, molecular modeling became more accessible with the widespread use of personal computers and graphical interfaces (GUI). From the 1990s onwards, modeling of more complex molecular systems became possible thanks to high-performance computers and advanced software (e.g. GROMACS (8) and AMBER (9)).
Basic Concepts for Molecular Modeling:
Atoms and Molecules: Atoms are the building blocks of molecules. Molecules are formed when two or more atoms combine with chemical bonds. The structural arrangement of molecules determines their chemical properties.
Chemical Bonds: The bonds formed between atoms and hold molecules together determine the stability and function of the molecule. The strengths and dynamics of these bonds are analyzed in modeling processes.
Molecular Geometry: Molecular geometry describes the arrangement of atoms in space within a molecule. Bond angle, bond length, and the three-dimensional shape of the molecule directly affect chemical properties.
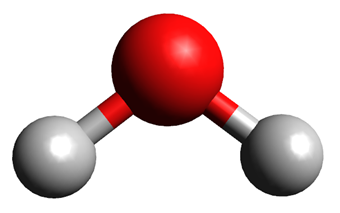
Potential Energy Surface (PES): The potential energy surface shows how the total energy of a molecular system changes depending on the different geometrical positions of the atoms. The PES determines the stable states and transition states of the molecule. This information is used to understand the energy barriers and possible pathways of chemical reactions.
Force Fields: Force fields are mathematical models used to calculate interactions between molecules. Force fields include bond strengths, angular tensions, and van der Waals forces between atoms. Commonly used force fields include AMBER (11), CHARMM (12), and GROMOS (13) and are the basis of molecular dynamics simulations.
Molecular Dynamics (MD) Simulations: Molecular dynamics simulations are simulations performed to understand how molecules move over time. The forces between atoms are used to predict the dynamic behavior of the molecule using Newton's laws of motion. Molecular dynamics also allows the study of the stability and flexibility of molecules over time.
The Importance of Computer Modeling
Computer molecular modeling allows us to understand the structures and interactions of molecules at the atomic level. In particular, modeling of complex biological systems can be done both faster and more economically with computers. Computer-aided modeling provides an alternative solution in cases where creating a physical experiment set is costly and time-consuming, thus speeding up processes and reducing costs. In addition, processes that are difficult or impossible to observe with experimental methods can be understood with computer simulations at the molecular level. Because computers allow us to reach details that cannot be reached in laboratories by simulating the interactions of millions of particles at the atomic level. This method is used in many areas, especially drug discovery (14), protein structure analysis (15), enzyme engineering (16), chemical reaction dynamics (17), etc.
References
1. Frank Vewinger, Bruce W. Shore, Klaas Bergmann. (2010). Chapter 3 - Superpositions of Degenerate Quantum States: Preparation and Detection in Atomic Beams, Pages 113-172, https://doi.org/10.1016/S1049-250X(10)05808-8
2. Terrell L. Hill; On Steric Effects. J. Chem. Phys. 1 July 1946; 14 (7): 465. https://doi.org/10.1063/1.1724172
3. Tamar Schlick. (2010). Molecular modeling and simulation: an interdisciplinary guide 2nd edition. Springer.
4. Nicholas Metropolis, Arianna W. Rosenbluth, Marshall N. Rosenbluth, Augusta H. Teller, Edward Teller; Equation of State Calculations by Fast Computing Machines. J. Chem. Phys. 1 June 1953; 21 (6): 1087–1092. https://doi.org/10.1063/1.1699114
5. B. J. Alder, T. E. Wainwright; Phase Transition for a Hard Sphere System. J. Chem. Phys. 1 November 1957; 27 (5): 1208–1209. https://doi.org/10.1063/1.1743957
6. H.M. Berman, J. Westbrook, Z. Feng, G. Gilliland, T.N. Bhat, H. Weissig, I.N. Shindyalov, P.E. Bourne, The Protein Data Bank. (2000). Nucleic Acids Research 28: 235-242 https://doi.org/10.1093/nar/28.1.235.
7. McCammon, J., Gelin, B. & Karplus, M. Dynamics of folded proteins. Nature 267, 585–590 (1977). https://doi.org/10.1038/267585a0
8. Van Der Spoel, D., Lindahl, E., Hess, B., Groenhof, G., Mark, A. E., & Berendsen, H. J. (2005). GROMACS: fast, flexible, and free. Journal of computational chemistry, 26(16), 1701–1718.
9. R. Salomon-Ferrer, D.A. Case, R.C. Walker. (2013) "An overview of the Amber biomolecular simulation package." WIREs Comput. Mol. Sci. 3, 198-210.
10. Marcus D Hanwell, Donald E Curtis, David C Lonie, Tim Vandermeersch, Eva Zurek and Geoffrey R Hutchison; “Avogadro: An advanced semantic chemical editor, visualization, and analysis platform” Journal of Cheminformatics 2012, 4:17.
11. Wang, J., Wolf, R. M., Caldwell, J. W., Kollman, P. A., & Case, D. A. (2004).Development and testing of a general amber force field. Journal ofComputational Chemistry, 25(9), 1157–1174. https://doi.org/10.1002/jcc.20035
12. Huang, J., & MacKerell, A. D., Jr (2013). CHARMM36 all-atom additive protein force field: validation based on comparison to NMR data. Journal of computational chemistry, 34(25), 2135–2145. https://doi.org/10.1002/jcc.23354
13. Reif, M. M.; Hü nenberger, P. H.; Oostenbrink, C. J. Chem. Theory Comput. 2012, 8, 3705−3723.
14. Akash, S., Bayıl, I., Hossain, M. S., Islam, M. R., Hosen, M. E., Mekonnen, A. B., Nafidi, H. A., Bin Jardan, Y. A., Bourhia, M., & Bin Emran, T. (2023). Novel computational and drug design strategies for inhibition of human papillomavirus-associated cervical cancer and DNA polymerase theta receptor by Apigenin derivatives. Scientific reports, 13(1), 16565. https://doi.org/10.1038/s41598-023-43175-x
15. Dai, L., & Zhou, Y. (2011). Characterizing the existing and potential structural space of proteins by large-scale multiple loop permutations. Journal of molecular biology, 408(3), 585–595. https://doi.org/10.1016/j.jmb.2011.02.056
16. Richter, F., Blomberg, R., Khare, S. D., Kiss, G., Kuzin, A. P., Smith, A. J., Gallaher, J., Pianowski, Z., Helgeson, R. C., Grjasnow, A., Xiao, R., Seetharaman, J., Su, M., Vorobiev, S., Lew, S., Forouhar, F., Kornhaber, G. J., Hunt, J. F., Montelione, G. T., Tong, L., … Baker, D. (2012). Computational design of catalytic dyads and oxyanion holes for ester hydrolysis. Journal of the American Chemical Society, 134(39), 16197–16206. https://doi.org/10.1021/ja3037367
17. Grimme S. (2019). Exploration of Chemical Compound, Conformer, and Reaction Space with Meta-Dynamics Simulations Based on Tight-Binding Quantum Chemical Calculations. Journal of chemical theory and computation, 15(5), 2847–2862. https://doi.org/10.1021/acs.jctc.9b00143
Comments